Q&A with Dr. Neil Bruce
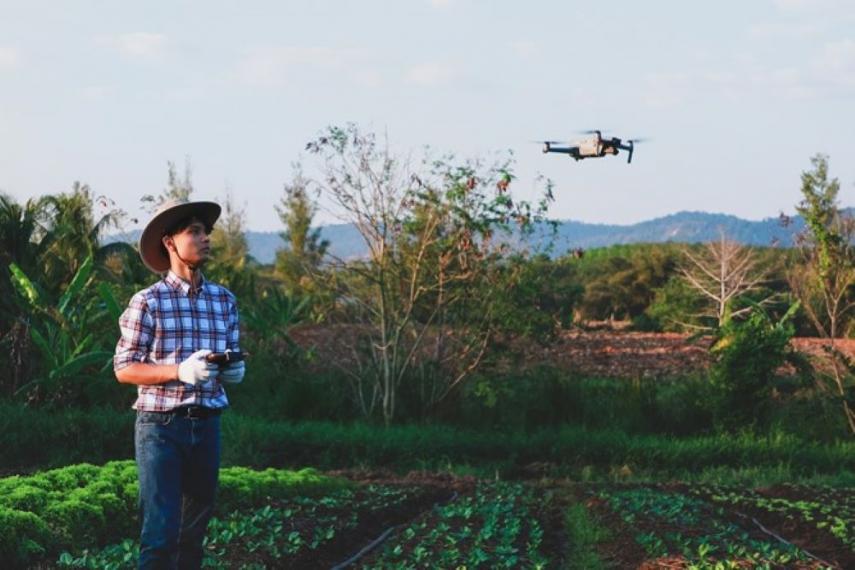
Dr. Neil Bruce’s research in computer vision has application in industries such as agriculture.
Self-driving cars. Virtual Assistants. Facial recognition. These technologies stem from a sub-field of machine learning in Artificial Intelligence (AI) called deep learning. Like a human brain, deep learning involves algorithms that mimic neural networks and can solve complex problems such as natural language processing or image classification. We spoke with Dr. Neil Bruce from the School of Computer Science about his research using deep learning to study computer vision and its widespread applications such as self-driving cars, medicine, agriculture, and manufacturing.
When did you join the School of Computer Science at the University of Guelph?
I joined the School of Computer Science at the University of Guelph in July of 2020.
Please describe your research focus.
My research focus involves the application of deep neural networks to dealing with various types of data. My work has a strong emphasis on visual media including photos and video. I explore solutions to issues in computer vision, deep-learning, and human perception. I am also especially interested in perceptual aspects of visual media and associated algorithms, including relating human neuroscience to artificial neural networks.
What do you see as the most exciting future applications for computer vision?
Some of the more obvious applications include things like self-driving cars, or diagnostics in medicine. However, I believe that progress in computer vision will extend into many other domains from agriculture to manufacturing to analysis and creation of new media.
Your research involves “explainable AI.” What does that term mean?
To me explainable AI refers to the capacity to understand how a complex AI solution reaches its conclusions or produces its output. In other words, humans can understand the path that a computer took to make its decision.
AI systems are becoming increasingly complex, and there is a real challenge in producing such explanations. Often, the system takes an action, and we don’t know how or why it occurred. We can try to relate outputs to the input of the system and attempt to draw inferences, however, I believe that truly explainable AI is going to require new ways of thinking and techniques to address this challenge with a greater level of maturity.
What is a recent research project/initiative that you are especially excited about?
One project my group is working on is what information neural networks are using to make decisions about images and what they contain (e.g. cat vs. dog). This research can be done by analyzing the objects in the image themselves, but very often other clues such as the background content of the image allow the system to perform well without truly forming a deep model of what objects look like.
Explicitly separating factors such as texture and shape allow for this relationship to be better understood and networks can be trained to be more shape-focused rather than texture-focused, which we’ve shown leads to greater robustness (consistently accurate in the face of errors or unforeseen circumstances).
Are you currently looking for undergraduate, graduate, or postdoctoral students?
I am always on the lookout for talented undergraduate, graduate, and postdoctoral trainees.
Dr. Neil Bruce is an Associate Professor in the School of Computer Science