Q&A with Ayesha Ali
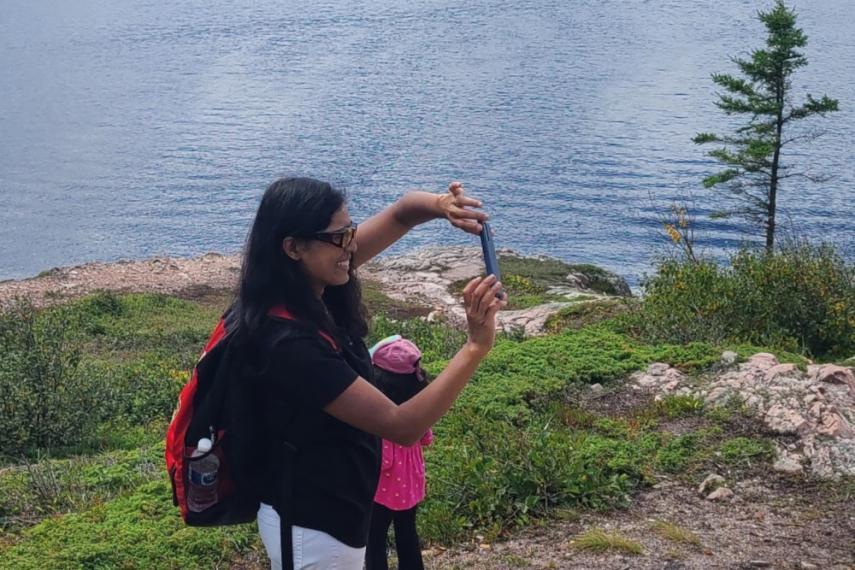
We spoke to Dr. Ayesha Ali from the Department of Mathematics and Statistics about her research on graphical models and its applications to agriculture and health.
When did you join the Department of Mathematics and Statistics at the University of Guelph?
I joined the Department in 2006. I had been teaching in the Department of Statistics and Applied Probability at the National University of Singapore and was looking to come back to Canada when this opportunity arose. My research roots lie in theoretical characterizations of graphs, and I loved that folks in our Department at U of G are largely focused on applied problems, often stemming from collaborations on campus. Aside from wanting to see the leaves change colours on the trees, I was excited to work on application driven problems. More recently, I have been involved in the new Master of Data Science program and in creating a community for these largely international students.
Could you tell us a bit about your research focus?
Graphical models provide a way to represent complex natural processes using networks, with nodes and edges, such that edges encode statistical information about how the nodes in the graph are related to each other. Sometimes the nodes represent variables in that process (e.g., genes in a gene network) and interest lies in understanding how nodes are related to each other and which edges are missing (structure learning). Recently, my work has been used to identify which genes are strong candidates to select for in an animal breeding program to reduce boar taint. If we have a sense of the structure of the underlying graph, then we can exploit the structure of the graph in estimating model parameters within a regression framework.
Other times, the nodes in a graph are the actors in some process (e.g., plants and pollinators in a pollination network) and interest lies in modelling why certain actors are interacting with each other (edge prediction). As a member of the Canadian Pollination Initiative, we developed models that used plant and pollinator traits to understand which traits may promote or inhibit certain plant-pollinator interactions. We assume each individual pollinator selects a floral species to interact with according to a multinomial distribution, where the probability of interaction depends on the pollinator species and floral species traits.
Part of your research focuses on genetic selection in livestock to improve overall animal health, can you explain the advances you are making?
Genetic selection uses gene expression data to select animals for mating that will either improve animal health, such as in the boar taint example (to avoid castration in young males), or to improve the animal product (e.g., produce milk with optimal fatty acid compositions). More recently, I’ve forayed into lipidomics, the study of lipids in cells, tissues and organs, for Quaker parrots to develop methods to detect differences in lipidomic profiles of healthy parrots and those suffering from dyslipidemias – an imbalance of lipids such as cholesterol. The challenge here is that we have high dimensional ‘omics’ type data, but very small samples sizes to work with.
Your research involves bootstrapping in longitudinal studies. Can you tell us how bootstrapping works and how you use it?
Suppose we have data that arose from a complex process, such as following subjects over time to observe when cancer survivors may relapse but there is a fraction of the survivors who will never relapse (i.e., those cured). It is challenging to estimate parameters suitable for this type of data and standard errors are not readily available. Bootstrapping allows us to compute standard errors and quantify the uncertainty in our estimation.
Bootstrapping involves simulating datasets from observed data through resampling. The simplest example is to sample observations from the data with replacement. If enough datasets are generated, the parameter of interest (e.g., average) can be computed from each simulated dataset and then standard errors, confidence intervals or hypothesis tests can be constructed for the parameter estimate.
What is a recent research project/initiative that you are especially excited about?
I have two projects I am excited about, one on crop-land use and the other on modelling gut bacteria. The former application involves using machine learning approaches to understand which land, soil and climate variables make a geographical region suitable for growing a given crop, and then trying to understand which regions of Canada will remain or become suitable in the future, within the context of climate change. The latter application involves modelling a gut-bacterial interaction network and trying to test for differences in gut composition between populations. Specifically, we will look at data from US immigrants and will apply our method to understand how their gut compositions may change as they become more acclimated to US culture and diet.
Are you currently looking for undergraduate, graduate, or postdoctoral students?
Yes, I am seeking a postdoctoral student to extend the plant-pollinator work, but within the broader context of ecosystem services, as well as Masters and PhD students.
Dr. Ayesha Ali is a Professor of Statistics in the Department of Mathematics and Statistics.