Bahram Gharabaghi
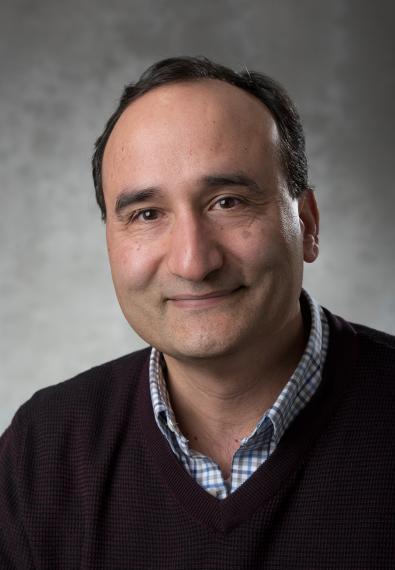
Instrumentation
A range of Standard Laboratory Testing and Field Monitoring equipment for both surface and groundwater water quantity and quality; specialized computers and software packages for data analysis and modelling.
Capabilities
We install real-time monitoring stations to study the movement of water and pollution within our surface and groundwater systems. We use monitoring data for model calibration and to study the fate and transport of pollution to develop better management policy guidelines and standards.
Education and Employment Background
Dr. Bahram Gharabaghi received his PhD in Water Resources Engineering from the University of Guelph in 1999. From 1999 to 2001 he held a position as a Postdoctoral Fellow in the School of Engineering at the University of Guelph. He then worked as a Hydrologist at Golder Associates, Mississauga, Ontario from 2001 to 2003. In 2003, Gharabaghi rejoined the School of Engineering at the University of Guelph where he is now a full professor.
Research Themes
Gharabaghi’s research goals align with the United Nations 2030 Agenda for Sustainable Development, focusing on improving the accuracy and reliability of modelling design tools and engineering decision support systems for managing urban water infrastructure in the face of challenges posed by rapid population growth and climate change. This is crucial for protecting our precious water sources from further degradation and devising effective solutions for restoring impaired water bodies (rivers, lakes, and groundwater). He takes pride in having had the honor of mentoring twenty-five master's students, fifteen doctoral candidates, and seven postdoctoral fellows to completion in the last two decades. Alongside his students, Dr. Gharabaghi has co-authored over 250 peer-reviewed scientific articles, over 50 conference papers, and 9 book chapters.
In Canada, we apply millions of kilograms of road salt each winter that cause salinization of our drinking water sources and harm aquatic life in urban streams and freshwater lakes, our research team has focused on developing algorithms for precision salt application using advanced machine learning methods to achieve the desired road safety with less adverse environmental effects.
Identification and Protection of Salt Vulnerable Areas: One of the primary areas of research in my lab is on the topic of road salt management. In cold-climate regions like Canada, Northern United States, and Europe, road maintenance authorities allocate significant annual funds toward ensuring road safety, which is particularly important during the winter season from November to April. Winter weather conditions, including air temperature, precipitation, and pavement surface temperature, are critical factors in determining the severity of winter storms and the accumulation of snow and ice on roads. Snow and ice build-up can decrease tire-road traction and increase the risk of accidents. To maintain safe road conditions and minimize the risk of serious car crashes during major winter storms, road authorities often use de-icing salts on road surfaces. However, the high chloride levels in the melted ice and snow runoff from roads can have adverse environmental impacts, particularly in salt vulnerable areas. The main goal of this research is to develop algorithms for precision salt application using advanced machine learning methods to achieve the desired road safety with less adverse environmental effects. Recent publications of our team on this topic, include: Radosavljevic et al. (2024); Safadoust et al. (2024); Tabrizi et al. (2022); Salek et al. (2018); Trenouth et al. (2015). Betts et al. (2014 & 2015); Perera et al. (2013). We have also focused on urban stormwater management tools, investigating the ecological effects of urbanization on fisheries and streams polluted by sediments and heavy metals. Our team has created models to predict and mitigate contaminants, safeguarding delicate ecosystems.
Urban Stormwater Management: One of the main research focus areas is on urban stormwater management. In particular, we investigated the thermal impacts of urbanization on cool and cold water fisheries and heavy metal contamination of urban streams. Stormwater management systems are often highly susceptible to thermal changes. Furthermore, discharging to temperaturesensitive receiving streams can have detrimental effects on ecology and water quality. Our research team has investigated the contaminants introduced to urban systems to predict heavy metal contamination from highway runoff. We have developed prediction models to design roadside ditch treatment systems to mitigate potential contamination to environmentally sensitive areas. Possible treatment systems were evaluated to assess their potential to protect these areas, such as by various filter medium introduced to stormwater swales, and the implementation of compost biofilters. This research allows for potentially harmful impacts of urban stormwater on sensitive environments to be accurately predicted and potentially mitigated. Recent publications include: Stajkowski et al. (2021 & 2023); Panjabi et al., (2021); Soltani et al. (2021); Jahanfar et al. (2020); Binns et al. (2019). Moreover, we have dedicated efforts to stream flow and water quality modeling, employing diverse machine learning tools to enhance assessment and restoration methods, improving our understanding of water resource engineering challenges.
Stream Flow and Water Quality Modelling: My research team have investigated the application of advanced machine learning tools, such as the adaptive neurofuzzy inference systems (ANFIS), support vector machines (SVM), artificial neural networks (ANN), and geneexpression programming (GEP) to develop new modelling tools and provide insightful contributions that have advanced the science of stream assessment and restoration. These models provide improved prediction capabilities due to their ability to create a relationship between the most influential input parameters and the desired output. Novel insight into common water resource engineering problems has been brought to light due to the combination of a strong understanding of the problems driving factors and by using machine learning techniques. Recent publications include: MacKenzie et al. (2022 a,b); Bonakdari et al. (2020); Milukow et al. (2019). More recently, our research team has centered our focus on constructing advanced predictive models to tackle complex environmental engineering problems with rapid spatial and temporal variabilities using available networks of real-time monitoring and remote sensing data. We have utilized cutting-edge deep learning architectures to address these multifaceted challenges more accurately and efficiently. Our investigations have included graph-based models that leverage data structures to inform deep learning applications, particularly in studying time-series spatiotemporal phenomena.
Graph-based Machine Learning Models: Our research delved into the implementation of graph-based models across diverse spatiotemporal scenarios in various studies. These models, as detailed in publications by Oliveira Santos et al. (2023a, 2023b, 2023c, 2023d), leverage graph structures to gain deeper insights into the inherent data structure. By integrating graphs with Deep Learning (DL) architectures, we achieved top-tier results in time-series spatiotemporal applications. The utilizationof a sample and aggregate graph framework facilitated the learning of embedding functions that can generalize to unseen nodes within the graph dataset. Notably, the Graph Attention Network (GAT) introduced by Oliveira Santos et al. (2023d) excels in discerning varying degrees of importance among neighboring nodes, employing an attention mechanism to process relevant parts of input data effectively. We have developed hybrid models that combine various deep learning techniques to enhance predictive capabilities, analyzing data both temporally and spatially for better outcomes.
Hybrid Machine Learning Models: Our endeavor has led to the development of hybrid models that combine different deep learning approaches for enhanced performance. For instance, Zhang et al. (2022) introduced a hybrid model merging Long Short-Term Memory (LSTM) with an attention mechanism, enabling the model to process time-series data efficiently while focusing on salient information within the input sequence. Additionally, the work by Tabrizi et al. (2021) integrated Convolutional Neural Network (CNN) and LSTM models to harness the strengths of each paradigm, allowing the model to analyze temporal and spatial aspects of the dataset simultaneously and improve overall performance. Our exploration of the Group Method of Data Handling (GMDH) models has evolved to incorporate more intricate data processing through self-organizing neural networks, overcoming limitations of traditional approaches.
Highlights
- Natural Sciences and Engineering Research Council of Canada, Discovery, Alliance, 2020-present
- Member, Professional Engineers of Ontario, 2002–present
- Lake Simcoe Science Advisory Committee Member, 2010–present
- Member, Road Salt Management Working Group of Environment Canada, 2010–present
Media Coverage
Lake Simcoe Science Committee
- Ontario Ministry of the Environment: Lake Simcoe Science Committee