Matthew Demers
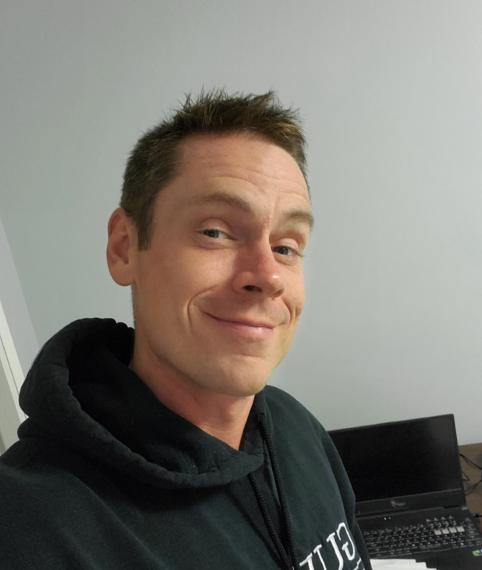
Find Related People by Keyword
Education and Employment Background
Dr. Matthew Demers received his PhD from the University of Guelph in 2012. Between 2012 and 2013, he was a postdoctoral fellow at the University of Guelph, where he worked to emphasize course development and delivery for mathematics and engineering students. Demers is currently an Assistant Professor of Mathematics in the Department of Mathematics and Statistics at the University of Guelph.
Research Themes
Demers’s research background is in the mathematics of images, largely focused on fractal-based methods in imaging, driven by iterated function systems (IFS) and the collage theorem. An IFS is a collection of contraction mappings. With that collection, we may form a special (Hutchinson) operator which possesses a unique attractive fixed point that possesses fractal properties. Demers currently focuses his research on the scholarship of teaching and learning, with a goal of better understanding math preparedness for incoming students with the aim of improving course delivery.
Demers has supervised many fantastic undergraduate student projects with topics including applications and extensions of IFS; modelling using ODEs and PDEs; traffic flow problems; cellular automata; and applications of genetic algorithms to a variety of problems. Demers has worked extensively now with two master's students. Key areas of focus in this work includes:
- Applications and Extensions of Iterated Function Systems. Essentially, the problem is as follows: "Given a target set, can we find an IFS whose attractor is 'close' to that target?" To approach this problem, we constructed a means of creating large sets of randomly generated fractal images. We then used this set of images as a training library for a convolutional neural network. With this neural network, we aimed to find the parameters defining a new IFS that might resemble that original target set.
- Extension of a COVID-19 epidemiological model to multiple-wave modelling in Ontario. Given a system of ODEs describing disease spread in the initial wave of COVID-19 occurring in China, we worked to apply this model to the situation since the beginning of the pandemic in Ontario over multiple waves. Involved in this work was modifying an existing model by adding complexities and time-dependence to multiple parameters. With this extended system, we used a "home-brewed" genetic algorithm to determine the optimal values of various parameters in the system to reflect the situation most accurately in Ontario. A goal of this work is to determine which control measures had the greatest impact in the trajectory of the disease spread over time.
Highlights
- The Last Lecture, Faculty Presenter, University of Guelph, 2017 and 2021
- Distinguished Professor Award for Innovation in Teaching, University of Guelph Faculty Association, 2015
- Professor of the Year, Engineering Society, Every Year Annually from 2012 through 2020
Media Coverage
Awards and Accolades
Learning Resources