Wenjing Zhang
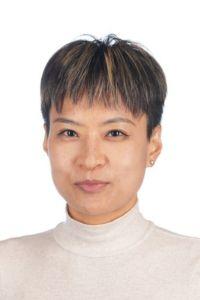
Find Related People by Keyword
Education and Employment Background
Dr. Wenjing Zhang received her Ph.D. in Computer Science from the University of Guelph in February 2024. From March 2024 to July 2024, she held a position as an adjunct professor in the School of Computer Science at the University of Guelph. In August 2024, she joined the School of Computer Science at the University of Guelph where she is now a tenure-track assistant professor.
Research Themes
Wenjing’s research interests are broadly centered around cybersecurity, Artificial Intelligence (AI), and Machine Learning (ML), with a particular emphasis on the interdisciplinary research of security, privacy, and machine learning. Her work is twofold: firstly, she utilizes machine learning techniques to study security and privacy. Secondly, she develops secure and privacy-preserving machine learning models. Key areas of her research focus include:
1. Security in AI and ML: Models in AI and ML are increasingly vulnerable to security threats aimed at compromising their functionality. These threats include poisoning attacks that corrupt training data, evasion attacks using adversarial examples to mislead models, and prompt injection attacks that manipulate inputs to large language models (LLMs). Wenjing’s team is highly interested in to developing robust defenses to secure AI and ML models against such threats. Her team’s efforts include designing empirically secure defenses using ensemble methods to counter both unseen and adaptive attacks, creating provably secure defenses that balance rigorous security guarantees with optimal model performance, and developing defenses against prompt injection attacks, particularly for LLMs.
2. Model Privacy in AI and ML: Model privacy involves protecting internal parameters to prevent model theft, even when attackers lack direct access to the model’s architecture, parameters, or training data. This protection is crucial for preserving the intellectual property of high-value model providers who invest heavily in expertise, large datasets, hyperparameter optimization, and computing resources to develop these models. Wenjing’s team works to advance innovative techniques that protect the privacy of both model parameters and outputs. This includes designing training frameworks that enhances the privacy-utility trade-off by leveraging statistical and information-theoretical approaches, creating mechanisms that perturb model predictions to minimize information leakage, and implementing collaborative privacy-preserving training techniques that enable multiple stakeholders to train and utilize models without sacrificing privacy.
3. Data Privacy in AI and ML: AI and ML models trained on real-world data can inadvertently memorize and reproduce sensitive information, thereby compromising the privacy of both training and testing data. To address this challenge, Wenjing’s team focuses on leveraging generative models to develop synthetic data generation algorithms that minimize privacy leakage while accurately replicating the statistical properties of real data. To further enhance the utility of synthetic data, her team designs reinforcement learning-based feature selection algorithms that ensure the fidelity and diversity of synthetic training datasets. Additionally, Wenjing’s team is interested in developing privacy-preserving prompt engineering techniques for LLMs to protect testing data while improving inference quality and effectiveness.
Canada has made substantial investments in innovation and economic growth and continues to do so, ensuring its world-leading AI advantage now and for generations to come. Recently, the Canadian government announced a $2.4 billion investment in AI as part of Budget 2024 to build infrastructure and drive adoption. However, the economic potential of AI is limited by significant security and privacy challenges, as highlighted by the Office of the Privacy Commissioner of Canada on June 21, 2023. The Canadian government is taking steps to maintain leadership in AI and security; on May 22, 2024, the President of the Treasury Board of Canada released the first Enterprise Cyber Security Strategy, supported by a $11.1 million investment over five years from Budget 2024. The research goals of Wenjing’s team align with this strategy by developing secure and privacy-preserving defenses for AI and ML models, addressing key security and privacy concerns, and supporting Canada’s efforts to fully maximize the economic benefits of AI.