Fei Song
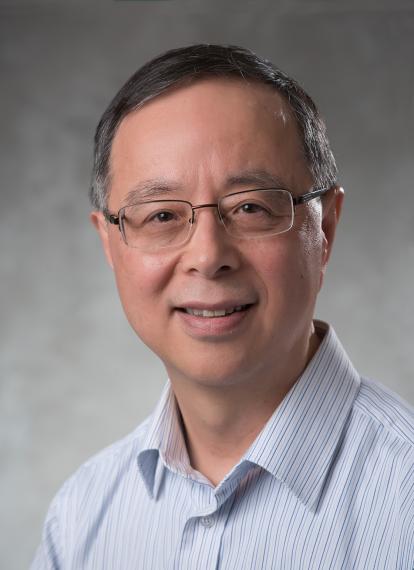
Find Related People by Keyword
Education and Employment Background
Dr. Fei Song received his PhD from the University of Waterloo. From 1990 to 1991 he held a position as a Research Associate at the University of Guelph. Song joined the School of Computer Science at the University of Guelph in 1991. In 1998, Song held a Visiting Scholar position at the University of Massachusetts at Amherst within the Center for Intelligent Information Retrieval in the Department of Computer Science. Song is now an Associate Professor at the University of Guelph.
Research Themes
Song’s research focuses on applying machine learning and artificial intelligence techniques to natural language processing, such as text classification, information retrieval, topic modeling, sentiment analysis, text summarization, text segmentation, and temporal analysis. Key areas of focus include:
- Solutions based on Artificial Intelligence (AI). In his early work, Song used knowledge representation to capture the meaning of a sentence and logical inferences to solve problems. He developed a processing model for the temporal analysis of narratives where initial temporal relations are extracted from the input and then tightened with rules in temporal logic. He also worked on the Dempster-Shafer theory and medical expert systems that can handle uncertainty in the inference process.
- Language processing tasks. In the late 1980s, the field of NLP started to change to the statistical paradigm where sequences of words (called N-grams) can be quantified with probabilities and Bayesian rule can be used to solve a wide range of language processing tasks. Following this paradigm, Song proposed a general language model for information retrieval that views the retrieval process as query generation based on the language models created for all the unigrams and bigrams of the corresponding documents. The related paper is well cited in the research community and is recognized in the Wikipedia page for “Language Model.”
- Topic modeling in statistical Natural Language Processing. Topic modelling can discover the underlying topics in a large collection of documents. Such hidden topics along with their proportions form a reduced representation of the given documents but still preserve the statistical relationships among data that are useful for many language-processing tasks such as text classification, novelty detection, and text summarization. Song and his team are exploring and extending the basic topic model, called Latent Dirichlet Allocation (LDA), by combining LDA with a syntax model so that both long-range dependencies among topics and local dependencies among words can be captured by the resulting model, called POSLDA.
- Sentiment analysis. Sentiment analysis (SA) classifies review documents into positive and negative categories and has many useful applications, such as analysis of product reviews and opinionated web search. Song and his team seek to apply machine learning techniques and different feature selection methods for sentiment analysis and compare their performance on both English and Turkish reviews.
- Convolutional Neural Networks (CNNs). CNNs are effective for many classification tasks. Song and his team are applying these models for different classification tasks, such as job title classification and key phrase extraction from food borne disease documents.
Highlights
- Precarn-OCE Grant, 2009
- Ontario Centres of Excellence Grant (OCE), 2006
- Natural Sciences and Engineering Research Council of Canada Discovery Grant, 1992, 1995, 2000