Gurjit Randhawa
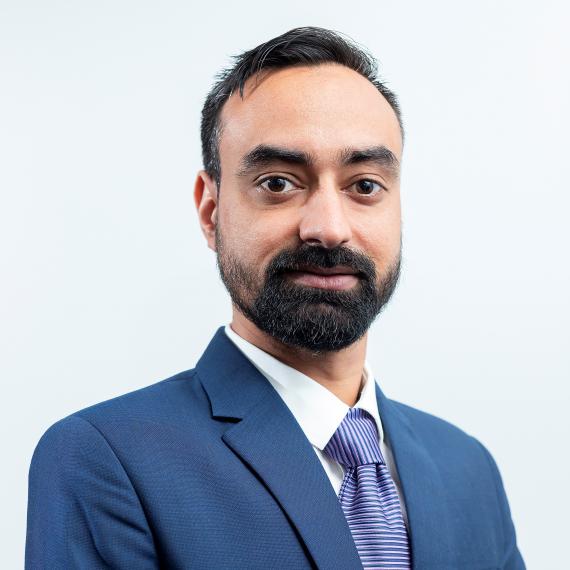
Find Related People by Keyword
Dr. Gurjit Randhawa received his Ph.D. and M.Sc. in Computer Science from the University of Western Ontario. After completing his Ph.D., he held a position as a Postdoctoral Fellow at the David R. Cheriton School of Computer Science at the University of Waterloo for a year. He then joined the School of Mathematical and Computational Sciences at the University of Prince Edward Island where he served as an Assistant Professor for three years. In 2024, Dr. Randhawa joined the School of Computer Science at the University of Guelph as an Assistant Professor.
My research focuses on applying machine learning to solve complex challenges across various domains, including genomics, bioinformatics, and precision agriculture. In bioinformatics, I am particularly interested in exploring genomic and mutational signatures through the analysis of genomic sequence data. Additionally, I have expanded my research to include the development of autonomous systems in precision agriculture to enhance farming efficiency and sustainability.
** Please note I have multiple positions available for M.Sc., Ph.D., and Postdoc candidates.**
Machine Learning in Genomics and Bioinformatics
Our work in bioinformatics centers around understanding genomic and mutational signatures by analyzing genomic sequence data. We employ machine learning techniques to address a range of challenges, including taxonomic classification, disease classification, and virus subtype identification. By leveraging advanced algorithms, we can uncover subtle patterns and correlations within large-scale genomic datasets, which are crucial for enhancing our understanding of biological processes and improving classification accuracy. For instance, we have studied the genomic signatures of extremophiles—organisms that thrive in extreme environmental conditions such as high temperature or pH—to understand how their unique adaptations are encoded at the genomic level. This research has provided valuable insights into the mechanisms of adaptation and holds potential applications in biotechnology and environmental management. Additionally, machine learning models have been applied to analyze bacterial genomes, providing insights into evolutionary relationships, and to classify viruses and diseases based on their unique genetic signatures. Through these applications, we aim to develop scalable, data-driven solutions that advance the field of genomics and contribute to a deeper understanding of genetic diversity and evolution.
Applications of AI in Precision Agriculture
In precision agriculture, our research aims to develop intelligent farming tools, particularly autonomous robots, that leverage machine learning for tasks such as disease detection, manual weeding, and precision spraying. We are currently working on an autonomous rover project designed to detect Potato Virus Y (PVY) infections in potato fields. The rover uses machine learning to identify infected plants, generate detailed infection maps, and support farmers in making timely decisions to prevent the spread of the virus. This approach not only boosts agricultural productivity but also reduces reliance on chemical treatments and promotes environmental sustainability. In the near future, we plan to extend this work to other crops, enhancing the versatility and impact of these technologies in various agricultural settings.
Highlights
NSERC-SSHRC Sustainable Agriculture Research Initiative Grant, 2024-present Natural Sciences and Engineering Research Council of Canada, Discovery Grant, 2020-present
Media Coverage
Artificial Intelligence in Precision Agriculture
- The Growers Magazine: The Artificial Intelligence eyes auto-labour
- CBC: This robot is using AI to help P.E.I. farmers detect potato viruses
Machine Learning in Bioinformatics