Q&A with Dr. Mihai Nica
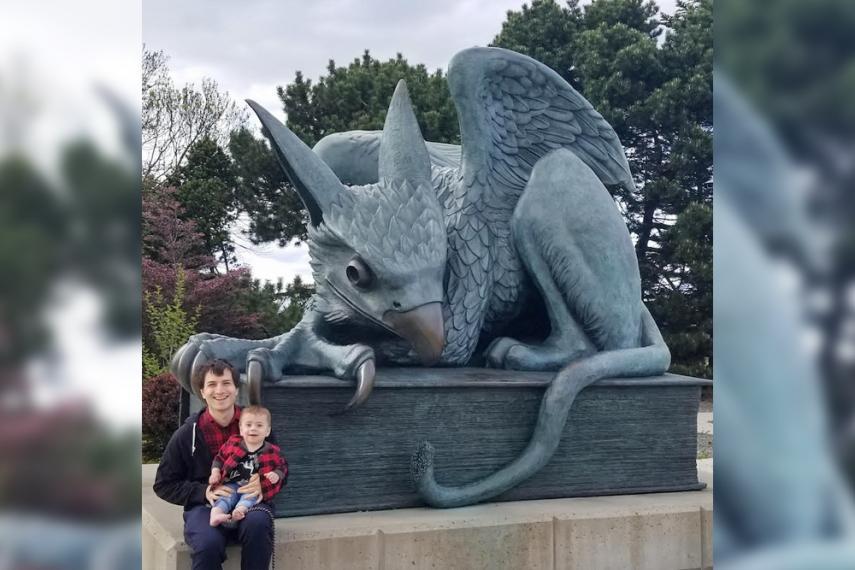
Dr. Mihai Nica shares his mathematical perspective on machine learning.
The first in our series of new faculty highlights, we chatted with Dr. Mihai Nica to learn more about his research program on neural networks, its exciting applications for our modern world, and his work solving the mysteries of modern machine learning.
When did you join the Department of Math and Stats at the University of Guelph?
I moved to Guelph from Toronto in April 2020 to begin my faculty position at U of G… Everyone knows the best time to move is at the height of a global pandemic!
Please describe your research focus.
I work on the mathematical theory of neural networks. In machine learning, neural networks are computing systems that were originally inspired by the behaviour of the human brain. I am interested in problems that center around understanding complex stochastic systems. A system is stochastic when one or more parts has randomness associated with it. Many machine learning algorithms are stochastic, and it is important to understand this randomness to develop predictive models.
I examine high-dimensional stochastic systems, meaning that the data are characterized by multiple dimensions. For example, when Artificial Intelligence (AI) is used for facial recognition, the computer learns to identify faces by drawing on many images and each image, in turn, has a resolution of multiple pixels—there are many dimensions to the data.
My research uses mathematical tools from theoretical probability, like the theory of random matrices and stochastic processes, to understand how these systems behave.
You work with Deep Neural Networks (DNNs). Explain what these are in simple terms and why they are important for the modern world?
DNNs are a type of function used in machine learning—it is an artificial neural network that has many layers. In the 2010s, the use of DNNs led to huge breakthroughs in a variety of tasks which were previously extremely difficult for computers, such as image recognition and game-playing Artificial Intelligence (AI). Because they can be used very generally, the use of DNNs is becoming more and more widespread in the modern world. Accurate voice recognition software like Amazon Alexa/Google/Siri are a great example of DNNs becoming part of our everyday lives.
Your work takes a unique approach to understanding AI technologies by applying mathematical analyses as opposed to having a computational focus. Why is it important to examine the math driving AI?
I like to think of the analogy to the historical roles of alchemy vs. chemistry. The original discovery of basic useful chemicals like gunpowder were discovered by alchemists: they discovered they could mix things and get exciting new results, but they weren’t very interested in why it worked.
Only later, the field of chemistry was developed that sought to understand what was going on scientifically. This advanced level of understanding led to incredible new discoveries, which the alchemists never could have achieved.
I see mathematical analysis of machine learning is a bit like that: my job is to dig under the hood of machine learning systems at a fundamental level. Developing a deeper understanding and new mathematical tools explaining how these systems work will open the door to future progress.
Are there research areas that you see opportunity for broader collaboration at U of G? What kinds of collaboration?
Absolutely! My work on the theory side complements nicely with people working on machine learning on the experimental side from the computer engineering and computer science departments. It’s been great meeting people in different disciplines on campus and I’m looking forward to broadening my collaborations here.
What is a recent research project/initiative that you are particularly excited about?
Some of my recent work is about understanding how the depth of a network effects network behavior. My work shows that the “aspect ratio” of the network, the ratio of how deep it is to how wide it is, is an important parameter that determines the network’s properties. It has always been a mystery in modern machine learning to explain why deep networks tend to outperform shallow networks, so this research is a step in the direction of explaining this difference.
Are you currently looking for undergraduate, graduate, or postdoctoral students?
Yes, all three levels! For undergraduate students nearing graduation, the collaborative master’s program in AI is a great program to get into machine learning at Guelph. I’m also teaching a fourth year/graduate course on a mathematical introduction to reinforcement learning in winter 2022 which could be an interesting course for interested students!
Nica applies math to the study of neural networks, artificial systems used to solve computational problems by imitating neurons in the human brain.