Deep Learning Offers Insight into Pipe Aging
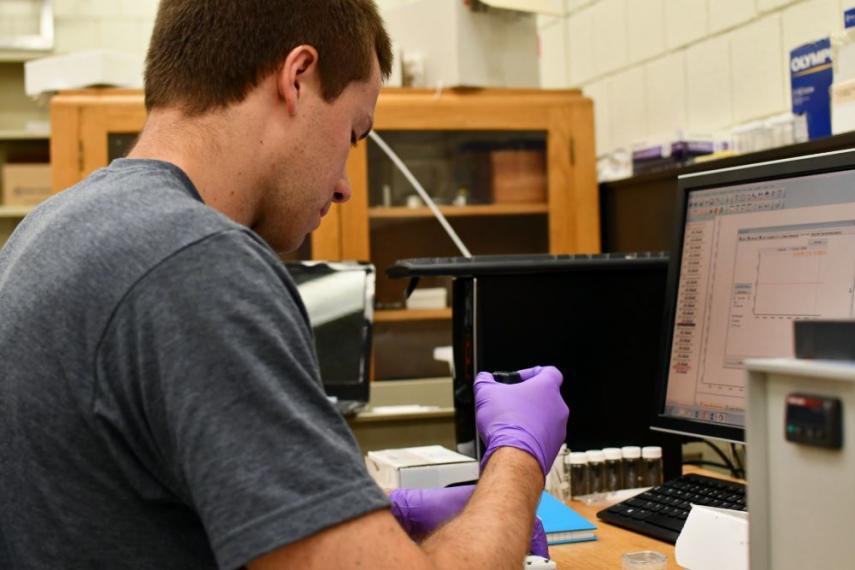
Research team uses machine learning to study the chemical changes in polyethylene pipe.
What do perfume quality control, forensic paint fragment analysis and plant variety identification have in common? They can all be analyzed or identified using infrared spectroscopy. Infrared spectroscopy, the analysis of how infrared light interacts with molecules, has been used as an analytical tool in multiple industries, such as food science, forensics, pharmaceuticals and petroleum. You can use this technique to obtain detailed information about the chemical composition of a material by analyzing how the material absorbs or reflects the infrared light. However, this information is difficult to extract from complex materials.
Overcoming infrared spectroscopy limitations
To overcome this constraint, statistical methods such as principal component analysis are often used to identify as much information as possible from the infrared spectroscopy data. However, there are limitations as to what information can be obtained. In machine learning, principal component analysis is considered one of the simplest learning mechanisms and works by summarizing a large dataset into a smaller one that still contains most of the information within the original larger one. With this overlap, machine learning approaches are increasingly being applied to spectroscopy data. One of these approaches is deep learning – a type of machine learning that uses artificial neural networks to simulate the network of neurons in the human brain so that the computer can learn and make decisions.
University of Guelph physics professor, Dr. John Dutcher, Research Associate Dr. Mike Grossutti and a team of students implemented a deep learning approach to study the spectroscopic and corresponding chemical changes that occur during the aging of a polyethylene pipe used for water transport and heating. They trained an artificial neural network on a data set of over 25,000 infrared spectra collected both in-house and at the Canadian Light Source of unused, in-service, cracked and aged pipes. They additionally performed a principal component analysis on the same data set for comparison.
Deep learning neural network able to identify cracks
Dutcher and his team found that deep learning outperformed principal component analysis when identifying chemical and compositional changes in polyethylene pipe. The neural network was able to learn and independently analyze the variance in the infrared spectroscopy data to accurately identify cracks in the wall of the piping. Utilizing deep learning could help with improving new pipe formulations as well as the planning of pipe replacements, ultimately reducing costs.
“This study shows how deep learning can enhance the analysis of spectroscopic data of complex materials,” says Dutcher. “By learning independent, interpretable and physically meaningful representations of spectroscopic data, deep learning can further broaden the applications of infrared spectroscopy to fields such as biomedicine and enable the extraction of more useful information from complex spectroscopic data sets.”
Dr. John Dutcher is a Professor in the Department of Physics and College of Engineering and Physical Sciences Research Chair in Novel Sustainable Nanomaterials.
This work was supported by a Natural Sciences and Engineering Research Council of Canada Collaborative Research and Development Grant. Part of this work was performed at the Canadian Light Source, a national research facility of the University of Saskatchewan, which is supported by the Canadian Foundation for Innovation, the Natural Sciences and Engineering Research Council, the National Research Council, the Canadian Institutes of Health Research, the Government of Saskatchewan, and the University of Saskatchewan.
Grossutti M, D’Amico J, Quintal J, MacFarlane H, Quirk A and Dutcher J. Deep Learning and Infrared Spectroscopy: Representation Learning with a β-Variational Autoencoder. J. Phys. Chem. Lett. 2022 Jun 21. doi: 10.1021/acs.jpclett.2c01328.