Engineering Grad Pioneering Machine Learning Research
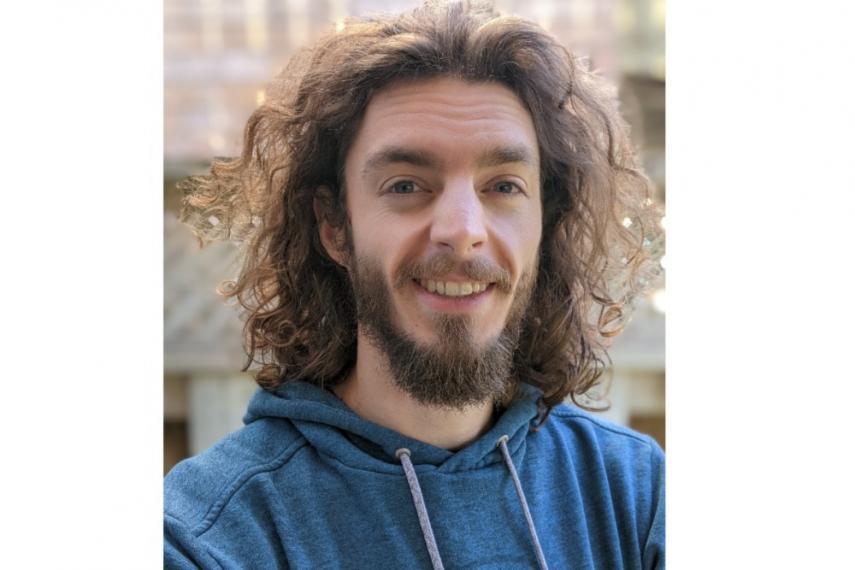
Dr. Terrance DeVries, a University of Guelph Engineering grad (PhD Engineering Systems and Computing ’21 and BEng Mechanical Engineering ’16) for computer vision and machine learning. We spoke to Terrance about being a pioneering machine learning research scientist at Luma Labs AI, his time at the University and his love of working with unique technologies.
Tell us about your work and your role with Luma Labs IA
I am a computer vision and machine learning research scientist on the founding team at Luma Labs AI. Luma is an early-stage startup whose mission is to make realistic 3D capture easy for everyone. We leverage recent developments in differentiable neural rendering and machine learning to create photorealistic 3D representations of objects and scenes using only images captured with our app. The end result can be thought of as a 3D photo where the camera can still look around and explore the scene even after the initial photo has been taken. You can see this technique through this demonstration using the University of Guelph Gryphon.
As a research scientist my main focus is to improve the overall quality and usability of our 3D reconstruction system. Often this consists of identifying failure cases that result in poor reconstructions and developing solutions for them or implementing new features and capabilities.
Tell us about a recent complex or interesting project you led
A core feature that I developed for the Luma capture system is the ability to automatically separate any scanned object from the background. In traditional photogrammetry and 3D reconstruction this separation of foreground and background can be a tedious manual process, so performing this operation automatically provides a significant time savings.
One of the main challenges with automatic object extraction is that the object can be anything, so the solution needs to be extremely general. I developed a robust solution which combines a variety of machine learning and computer vision techniques in order to ensure that the system always isolates complete, semantically meaningful objects (as opposed to randomly cutting things in half when it fails to identify the target object).
What do you enjoy most about your work?
I really enjoy working on cutting edge technology and seeing people’s reactions when they use our app to capture a scene. Most people aren’t aware of the capabilities of this technology yet and are often amazed when they see what they can create in 3 minutes of scanning with a phone.
Another aspect that I enjoy about my work is the variety of projects I get to work on. Since the company is a startup, I’m often working on something new each week (if not each day), which gives me the opportunity to learn a lot very quickly.
What motivates you to do the work that you do? Do you have any specific interests that drive you?
The technologies that I get to work on, differentiable rendering and machine learning, are both incredibly interesting and fun to work with. It feels like magic whenever things work well, and research in these areas is progressing at a breakneck pace and getting better all the time. It is all very exciting, and even a bit overwhelming.
Additionally, I really like seeing what different applications and creative uses everyone has for our technology and the app we’re creating. So far, we’ve already seen a wide variety of use cases, for example: capturing memories in 3D, bringing toy figurines to life in augmented reality, adjusting camera paths post-hoc for VFX, and reconstructing underwater ship hulls for visual inspection.
How did your education and research at U of G contribute to your journey after graduation?
In grad school I studied machine learning, specifically focusing on generative models. Generative models are a powerful type of machine learning model. You can take a large collection of data, such as images, train a generative model on the dataset, and then it can generate new, never before seen images from scratch.
One of the challenges with training these generative models is in collecting the data to train them. It requires a large effort to find relevant data, and to filter out things that may not be relevant to what you want the model to generate. One of my research projects during my PhD addressed this problem by demonstrating a way to automatically filter through images in order to produce a more useful dataset for training the generative model. Another project tackled the problem of training generative models to produce 3D scenes using only 2D image data for training. I hope to use ideas from both of these projects at Luma to enable the creation of generative models so that people can not only scan existing objects, but also create from scratch any 3D object that they can think of.
Looking back, what stands out to you the most about your experience at the University of Guelph?
For me what stands out is all of the networking opportunities that I had available while a grad student at U of G. My advisor Graham Taylor was (and still is) amazing at encouraging students to attend talks, summer schools and conferences, and also at facilitating internships and research collaborations. The networking that occurred during these events was invaluable, and I am thankful to have had the chance to meet and work with so many amazing people during my studies.