Q & A with engineering faculty member Dr. Eran Ukwatta
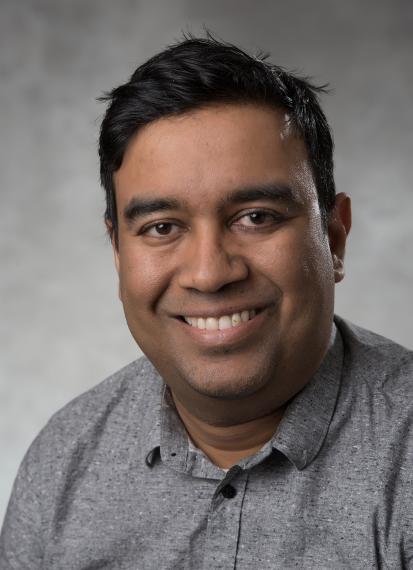
Working in the School of Engineering, Dr. Eran Ukwatta uses AI to improve human health. We spoke to him about his research on using artificial intelligence to interpret medical images to assist with diagnosis and prognosis of disease.
When did you join the School of Engineering at the University of Guelph?
I joined the School of Engineering at the University of Guelph in August 2018. Prior to that, I was an Assistant Professor (tenure-track) in Systems and Computer Engineering at Carleton University.
Could you tell us a bit about your research focus?
My research is focused on developing software tools that can automatically interpret medical images to assist with diagnosis and prognosis of disease. I primarily use deep learning methodologies (a branch of AI), which has transformative applications in human and veterinary medicine. My research finds practical applications in medical image segmentation (e.g., heart, liver, kidneys and prostate) and computer–aided detection of cancer in medical images, such as in magnetic resonance imaging, computer tomography, ultrasound and digital histopathology.
One of the many applications of your research is found in the field of medicine. Can you walk us through how you use machine learning to help develop diagnostic methods for detecting cancer?
Medical images have been a key part of most cancer screening programs for primary and secondary care. At the moment, most of the diagnostic work involves clinicians’ eye balling through the images to make a decision on diagnosis or treatment. The size of these images and number of images have evolved over time, so this process is very tedious, time consuming and subject to high operator variability. With the availability of large imaging datasets, we can now use machine learning techniques to harvest the patterns that are embedded within the data. To do this we use deep learning techniques in a supervised manner (e.g., like a teacher and student setup, where data is the teacher, and the AI model is the student) to train a classifier to perform certain tasks. These tasks can range from image segmentation to detecting tumours in medical images to predict a diagnosis.
Part of your research focuses on cardiovascular imaging applications for stratifying patient risk. Can you explain why you chose this focus and what you hope to achieve?
Cardiac disease is a leading cause of death around the world, and I chose this area to work as I believe that imaging coupled with the latest developments in AI may be leveraged to stratify the patient risk of arrhythmias. One approach for this is to create an image-based computational cardiology model of the patient and then to simulate electrical conduction of the heart to predict the occurrence of arrhythmias such as ventricular tachycardia, a life-threatening condition. With the availability of large annotated datasets there is also potential to use just AI to predict the risk of arrhythmias. My ultimate goal is to translate and transfer these technologies into clinical care to make a real impact on human lives.
What is a recent research project/initiative that you are especially excited about?
I am really excited about the neonatal brain imaging project that we are working on in collaboration with the Robarts Research Institute in London, Ontario. These are neonatal patients (under one month old) suffering from a potentially life-threatening condition called, intraventricular hemorrhaging. One of the main causes of that is the Hydrocephalus, which is an abnormal buildup of cerebral spinal fluid in the ventricles deep within the brain. Currently, 2D ultrasound is used for this diagnosis, but it is not very sensitive to the volumetric changes. We, however, are using 3D ultrasound to diagnose and monitor the growth of the ventricles in these children. Since 3D ultrasound images are big and very challenging to be segmented, we have developed fully automated AI-based methods to segment the ventricles and then quantify the volume of the ventricles. This allows us to conduct timed follow ups with these patients over time until the risk is low.
Are you currently looking for undergraduate, graduate, or postdoctoral students?
Yes. I am consistently looking for graduate and undergraduate students.
To learn more about Dr. Ukwatta's research you can visit his lab website.