Artificial Intelligence Improves Screening Accessibility for Liver Disease
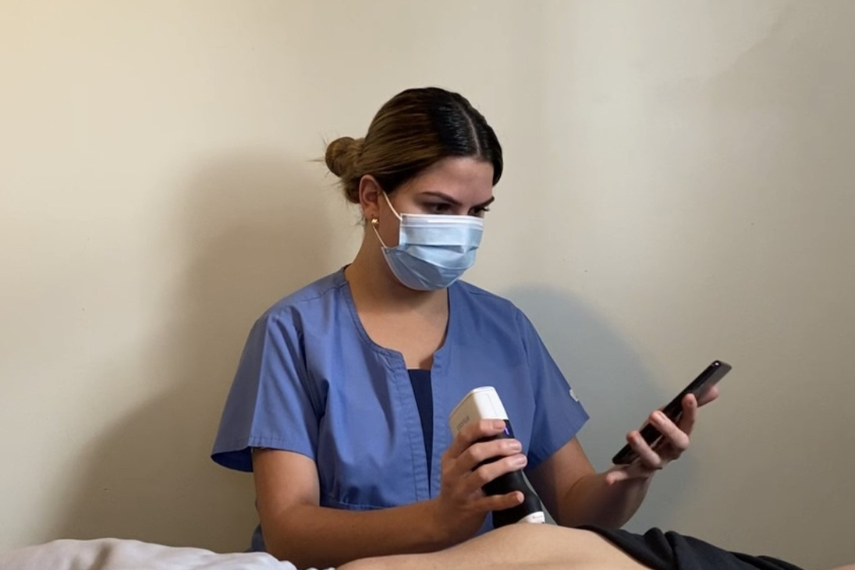
Researchers develop an automated method that can detect a prevalent liver disease at the primary care level.
A Global Health Concern
Non-alcoholic fatty liver disease (NAFLD) is a chronic liver condition that affects approximately 25% of the population worldwide. Early stages of NAFLD are relatively harmless and asymptomatic, but over time it can progress to serious liver diseases, such as cirrhosis. In these later stages of NAFLD, the damage to the liver is irreversible and very difficult to treat. This makes NAFLD concerning since patients are often unaware of the disease’s progression until it is too late.
Early detection of NAFLD can result in treatment to reverse the disease. Currently, NAFLD screening procedures, such as transient elastography, diagnostic ultrasounds, liver biopsies and magnetic resonance imaging (MRI), require highly trained personnel and expensive equipment. This limits the accessibility of NAFLD screening, particularly in regions with limited health care resources. The ability to implement screening at the primary care level, such as a physician’s office, may help to improve early NAFLD detection and treatment.
Making Screening More Accessible
Miriam Naim Ibrahim, an MA.Sc graduate from the University of Guelph’s School of Engineering, wants to develop a more accessible way to screen for NAFLD. Working with her former advisor, Dr. Eranga Ukwatta, an Associate Professor in the School of Engineering, and Oncoustics, an industry partner where Ibrahim is now employed, the team devised a method for using artificial intelligence and machine learning to screen patients for NAFLD.
A clinical study consisting of 478 patients was used to test their screening procedure. Ultrasound images were taken of the participant’s abdomen, from which an image of the liver was segmented– a method that divides an image into smaller subsections in order to process pieces individually. Small patches of the liver image were further isolated, and established machine learning algorithms were used to detect the presence of steatosis, the first stage of NAFLD. A binary (“yes” or “no”) response was then generated from the algorithm. Several different machine learning algorithms customized and optimized by the team were tested in order to identify the algorithm that best detected NAFLD. Responses were compared to results from a Fibroscan®, a transient elastography scan of the liver used in specialty level care for classifying the stiffness and attenuation of liver tissues, performed and interpreted by a qualified technician.
Results from the study demonstrated that early stages of NAFLD can successfully be detected using ultrasound images combined with machine learning algorithms. Positive cases were correctly identified at a rate of 95%, while negative cases were correctly identified at a rate of 82.5%. These success rates are comparable to standard practices, but with the advantage of being more cost effective and easy to handle. “Previous studies done with standard clinical ultrasound are not as portable and are a lot more expensive,” says Ukwatta. An additional benefit is that the screening outputs a “yes” or “no” result and does not require a highly trained ultrasound technician to interpret the scan, making it more accessible in primary health care settings. “All the images in the study were taken by staff, nurses, and students, who did not have a background in sonography, and received minimal liver ultrasound training for the study” explains Ibrahim. The algorithm’s pipeline is able to identify portions of the ultrasound image which contain liver tissue and discard images without liver visibility.
Extending the Technology
Ibrahim and Ukwatta plan to continue studying how artificial intelligence can be used in disease screening and detection. “Oncoustics is exploring software that can be applied to other liver diseases,” says Ibrahim. Ukwatta hopes to continue working with industry partners to investigate other potential biomedical applications of artificial intelligence.
This story was written by Carley Miki as part of the Science Communicators: Research @ CEPS initiative. Miki is a PhD candidate in the Department of Physics under Dr. John Dutcher. Her research focus is on understanding the forces and interactions between soft, sugar-based nanoparticles and how they differ when charged.
Funding Acknowledgement: This work was supported by The University of Guelph and MITACS.
Ibrahim, M. N., et. al. Automated fatty liver disease detection in point-of-care ultrasound B-mode images. J. Med. Imaging. 2023, 10 (3), 034505. doi: https://doi-org.subzero.lib.uoguelph.ca/10.1117/1.JMI.10.3.034505