eDNA Collection Gets a Tech Upgrade
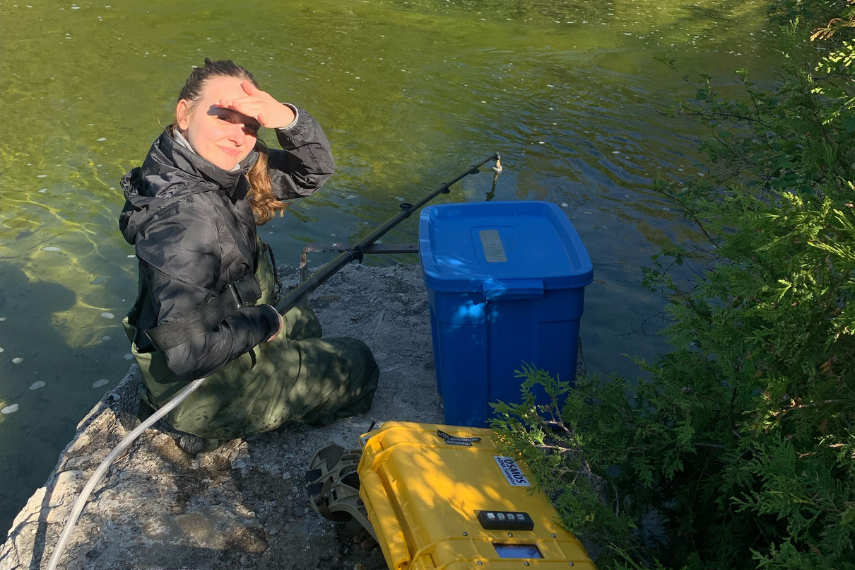
DNA is everywhere. It is in skin cells shed by snakes, in eggs released by spawning fish and even in droppings left by deer! These tiny traces of genetic material lingering in water, sediment, soil and air have a name—environmental DNA, or eDNA—and for biodiversity researchers, eDNA is an invaluable treasure trove of information.
This summer, computer science student Nikolett Toth teamed up with Drs. Jarrett Phillips, Daniel Gillis and Luiza Antonie from the University of Guelph’s School of Computer Science, as well as Dr. Robert Hanner from the Department of Integrative Biology. Together, they embarked on a project to enhance eDNA sampling using machine learning techniques.
The eDNA Degradation Problem
eDNA allows scientists to detect the presence of endangered species, track the spread of invasive pests and assess the health of entire communities and ecosystems.
Still, eDNA technology is not without its challenges. DNA, like all biological material, degrades over time, often in mere hours. This poses a significant challenge for researchers, as species might be falsely presumed absent from sites they inhabit, simply because their DNA degraded before it could be collected—no DNA, no detection.
Temperature and pH are two of the most significant variables affecting eDNA degradation rates, with higher temperatures and more acidic conditions causing genetic material to degrade more quickly. Additionally, factors such as the size of the environmental sample collected, UV exposure and microbial activity can also influence degradation.
Understanding the factors which affect eDNA’s persistence, and how they interact, is crucial if eDNA technology is to be adopted by government and industry as a viable conservation and monitoring tool.
Machine Learning to the Rescue!
Toth and Phillips propose applying association rule mining, a machine learning technique that discovers interesting relationships between variables in datasets, to better understand aquatic eDNA degradation rates. Their interdisciplinary collaboration with Gillis, Antonie and Hanner focuses on scanning eDNA datasets to identify variables linked to high eDNA concentrations. For example, these high concentrations might be associated with factors such as large sample volumes and low pH.
Quantitative measures, such as support, can be used to calculate the significance of associations between variables in datasets. From the strongest associations, rules can be generated to predict optimal conditions for eDNA sample collection.
“To our knowledge, association rule mining has never been applied to eDNA datasets before,” says Phillips. “We think it is a very promising technique to understand what combinations of conditions most affect eDNA, helping researchers collect environmental samples under the best possible conditions.”
Day in the Life
As a student researcher, part of Toth’s position involves eDNA sampling in rivers across Ontario. Using a device that resembles a long fishing rod, equipped with a cartridge at the end, she draws water through a filter where sediment, DNA and other matter accumulate. These filters are then taken to the lab for analysis to reveal the hidden genetic diversity within the water.
In addition to fieldwork, Toth spends a lot of time filtering and processing data to identify association rules that highlight patterns and correlations in eDNA detections. This analytical task is complemented by reading scientific literature to stay updated on the latest developments in eDNA research and machine learning. Each new piece of information supports the goal of improving biodiversity monitoring and protection.
A Promising Future
As the team continues to uncover new insights about the variables affecting sample concentrations, the potential for eDNA technology to revolutionize environmental monitoring and protection becomes increasingly clear. This project not only exemplifies the power of interdisciplinary collaboration but also highlights the promising future of combining computer science with biodiversity research to preserve our planet’s precious ecosystems!
This work is supported by an Undergraduate Research Assistantship (URA) to Drs. Daniel Gillis and Jarrett Phillips.