Machine-learning advances personal ads
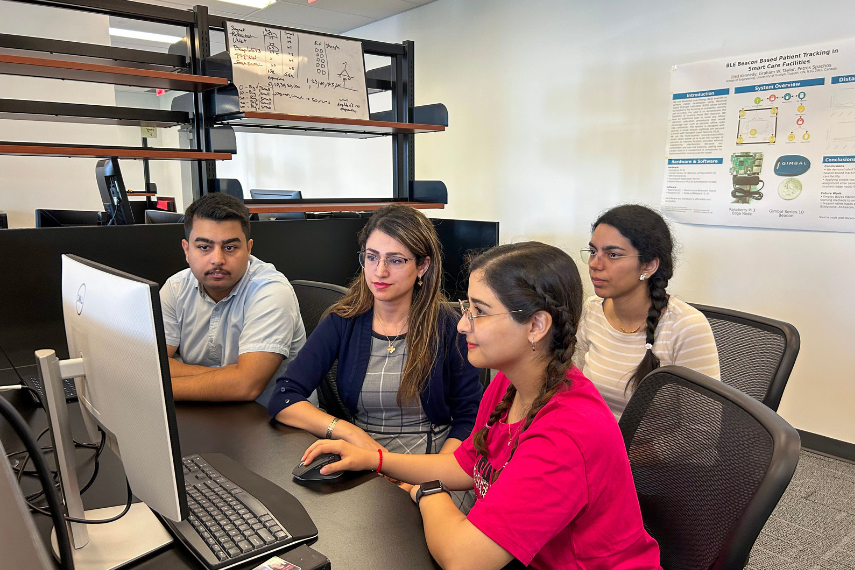
Current personalized advertising models have limitations
Traditional television commercials were designed to be broadcasted to wide audiences and general demographics, whereas the advertising we are experiencing today involves specific ads targeted to our social media feeds based on our behaviours and/or personal information. Accurate, customized ads are more engaging and useful to users as individuals, leading to higher engagement rates and potential increase in sales for the advertisers.
The specific ads on your social media feeds come from machine learning models that analyze users’ current or past interests expressed through their activity on social media (e.g., posts, re-posts and likes). Advertisers use future-interest prediction models to select the best candidates for a particular ad, and the existing framework behind these models assumes that a user’s current interests will be the same in the future.
What the current framework behind these models fails to acknowledge is that humans are dynamic, and we evolve and change the topics we are interested in over time. Also unaccounted for is topics that have yet to appear on the social media platform, termed “cold topics”, or topics that a user has never interacted with. Essentially, the current framework behind future-interest-prediction models does not factor in ‘temporality’, which can be thought of as the dynamic nature of people’s interests, how they can evolve over time and in response to world events.
Research from the University of Guelph’s School of Engineering (SOE) not only evaluates the effectiveness of the different variables that are involved in future-interest-prediction models, helping to create a better reference framework, but also explores the influence of temporality on the accuracy of these models.
New reference framework improves the targeting of social media ads
Dr. Fattane Zarrinkalam, an assistant professor in SOE, developed a learn-to-rank (LTR) reference framework for supervised machine learning to predict the future interests of social media users. This “Learning-to-rank” framework helps a future interest prediction model suggest the most relevant topic for a user. Using a real-world data set from Twitter, Zarrinkalam categorized users into high, medium, and low activity groups before testing the efficacy of the framework. The efficacy of each variable – while accounting for the influence of temporality – was tested over a set of “future-yet-unobserved topics”, which have not yet been observed on the social platform but are expected to emerge.
The LTR framework testing concluded that for accurate future interest prediction across all user activity groups, a user’s historical interests and the influence of temporality on those interests must be considered. Another powerful indicator for predicting future interests is the relevance of a user’s historical interests related to the “future-yet-unobserved” topics. To improve prediction accuracy for “cold topics”, the most beneficial variables are users’ influence, personality, and susceptibility to be influenced by friends’ interests. For low activity users, the popularity and freshness of a topic on social networks can strongly predict their future interests, displaying a clear popularity bias on social media platforms.
"Insights from this research have broad applications, including improving recommendation systems, enhancing user engagement, and optimizing content delivery on social networks,” said Zarrinkalam. “Industries that rely on understanding and predicting user behaviour, such as advertising, can gain significant value from these findings, enabling them to refine strategies and increase their impact."
Her future work plans to mitigate the influence of any biases on the accuracy of this future interest prediction framework.
Zarrinkalam F., Noughabi H.A., Noorian Z., Fani H., Bagheri E. Predicting users’ future interests on social networks: A reference framework. Inform. Process. Manag. 2024 May 1. doi: 10.1016/j.ipm.2024.103765